Unlock Yield by Addressing Behavior-Action Mismatches in Enrollment
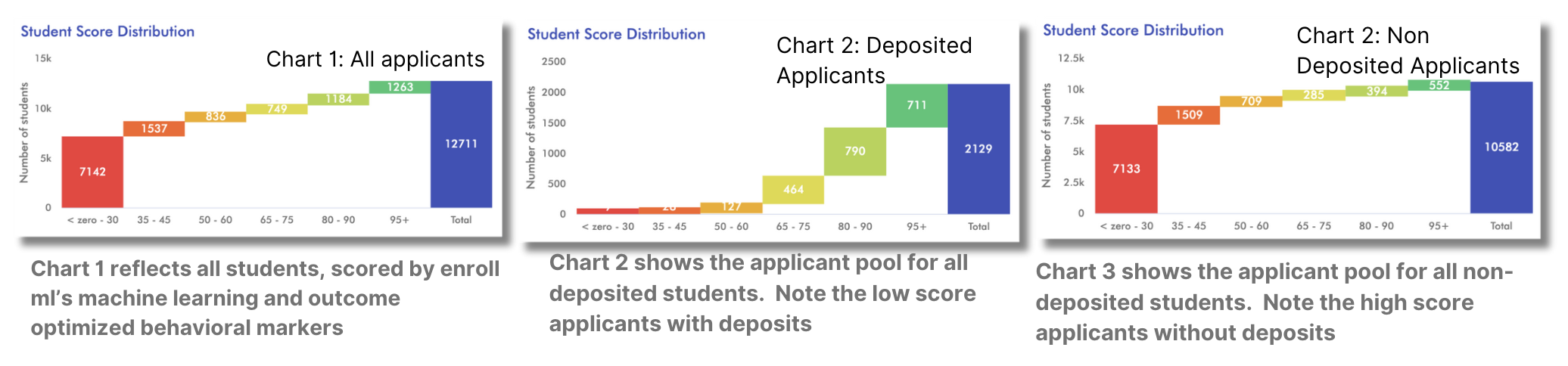
Introduction
In today’s competitive enrollment landscape, yield and retention hinge on data-driven precision. Traditional methods often fall short, relying on subjective judgment and missing critical engagement signals. enroll ml changes this by identifying behavior-action mismatches, helping admissions leaders spot opportunities and risks in real time for measurable impact.
Understanding Behavior-Action Mismatches
Behavior-action mismatches occur when engagement doesn’t align with commitment indicators, such as:
- High Engagement, No Commitment: High-potential students show interest but haven’t reached a commitment milestone, signaling a prime yield opportunity.
- Low Engagement, Commitment Met: Students with commitment indicators but low engagement, often an early indicator of melt risk.
Challenges with Traditional Methods
For many admissions teams, sifting through high application volumes and disconnected engagement data can feel overwhelming. Traditional models track disconnected behaviors that may correlate with enrollment but don’t provide enough depth to detect patterns. This often leads to delays or missed opportunities for timely intervention.
How enroll ml Closes the Gaps
enroll ml’s machine learning and Outcome Optimization Theory detect behavior-action mismatches in real time, transforming how admissions teams prioritize engagement:
- Multi-Marker Modeling: By analyzing an interconnected range of behaviors—engagement depth, timing, communication patterns—enroll ml precisely identifies high-potential, high-impact students.
- Automated Flagging and Prioritization: enroll ml flags behavior-action mismatches daily, eliminating time spent on manual record review. This prioritization empowers teams to re-engage at-risk students before melt occurs, turning data analysis into focused, real-time action.
Strategic Impact of Addressing Behavior-Action Mismatches
By automating prioritization, enroll ml can reduce counselor time spent on administrative tasks by up to 30%, freeing them to focus on high-impact engagement with top-yield prospects.
- Enhanced Yield: With immediate engagement, admissions teams lift yield by focusing on high-engagement students nearing commitment.
- Case in Point: Within days of launch, Columbia College Missouri identified and converted high-potential students previously overlooked, directly boosting yield by X%.
- Reduced Melt: Proactive outreach to at-risk students with commitment indicators minimizes last-minute withdrawals.
- Case in Point: Roosevelt University significantly reduced melt by re-engaging low-engagement depositors flagged by enroll ml, leading to X% decrease in summer melt.
Takeaway for Enrollment Leaders
Monitoring and responding to behavior-action mismatches directly impacts final yield outcomes. With enroll ml, admissions teams continuously identify actionable signals, strategically unlocking yield and reducing melt. Ready to turn data into yield? With enroll ml’s behavior-action insights, admissions leaders can unlock hidden yield potential and reduce melt, leading to X% gains in yield and X% reductions in time spent on administrative tasks.
Comments