From Profiles to Behaviors: Transforming Data Analysis in Enrollment Management
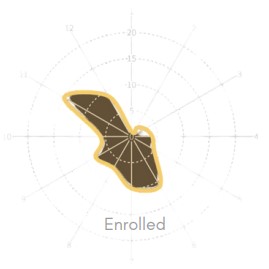
As we become increasingly reliant on large-scale data analysis in higher education, traditional methods of predicting student enrollment often fall short. Relying on static profiles and broad demographic data can lead to imprecise predictions and missed opportunities. Enter machine learning: a revolutionary approach that shifts the focus from static profile-based prediction to dynamic behavioral tracking, offering a more nuanced and effective way to manage enrollment.
Machine learning leverages real-time behavioral data, such as interactions with digital platforms, engagement with communication channels, and participation in events, to create a comprehensive picture of each student's journey. This shift allows admissions teams to move beyond generic profiles and understand the unique behaviors and preferences of individual students.
One of the key benefits of this approach is its ability to provide real-time insights, enabling institutions to react swiftly to changing enrollment environments. By tracking dynamic data points, machine learning models can identify patterns and trends that static profiles simply cannot capture. For example, traditional methods might classify students based on age, GPA, or geographic location. In contrast, machine learning can analyze the frequency of campus visits, the timing of application submissions, and engagement with digital content to predict enrollment likelihood more accurately.
This leads to more precise predictions and targeted engagement strategies, ensuring that high-potential students receive the personalized attention they need. Admissions teams can focus their efforts on students who are most likely to enroll, increasing efficiency and effectiveness. This targeted approach not only improves enrollment rates but also enhances the student experience by providing timely and relevant interactions.
Moreover, the use of machine learning in enrollment management can significantly enhance decision-making processes. Admissions teams are often overwhelmed with data, spending a considerable amount of time on manual data analysis and interpretation. Machine learning automates these processes, allowing for the swift analysis of complex datasets and freeing up counselors to focus on building relationships with prospective students.
This automation and optimization increase counselor capacity by over 30%, enabling more strategic use of their time. For instance, rather than sifting through piles of applications manually, counselors can rely on machine learning algorithms to highlight the most promising candidates based on their behaviors and engagement levels. This not only saves time but also ensures that no high-potential student slips through the cracks.
Additionally, the integration of machine learning into enrollment strategies allows for continuous improvement and adaptation. Machine learning models are designed to learn and evolve over time, incorporating new data and adjusting predictions accordingly. This means that as student behaviors and preferences change, the models can adapt, ensuring that institutions remain responsive to the latest trends and insights.
By embracing machine learning and behavioral tracking, institutions can transform their enrollment strategies, making them more adaptive, precise, and student-centered. This innovative approach promises to enhance decision-making, optimize resource allocation, and ultimately, improve enrollment outcomes. In a competitive higher education landscape, leveraging the power of machine learning can provide institutions with a significant advantage, ensuring they attract and retain the best-fit students.
Comments