657 Hours Behind A Screen

Admissions directors tend to look for outgoing, energetic, sometimes even effervescent personalities to be admissions counselors. We want someone who will connect easily with students, while still be relatable to parents. They need to work with a wide range of people from different backgrounds and unique goals.
When we succeed in a search, we have a new, optimistic, excited staff member ready to connect with students and make a difference in their lives.
Then, we literally tell them to sit down and figure out how pivot tables work for a third of their time in the office.
This is a real cause of the staff morale crisis - something we discussed in a webinar for Montana ACRAO last year.
.png)
Teege Mettille
Higher education professional with experience in admissions, enrollment, retention, residence life, and teaching. After working on six different college campuses, I'm excited to be consulting with a wide variety of institutions to better meet enrollment targets.I have been fortunate to serve as President of the Wisconsin
Leave a comment
Featured blog posts
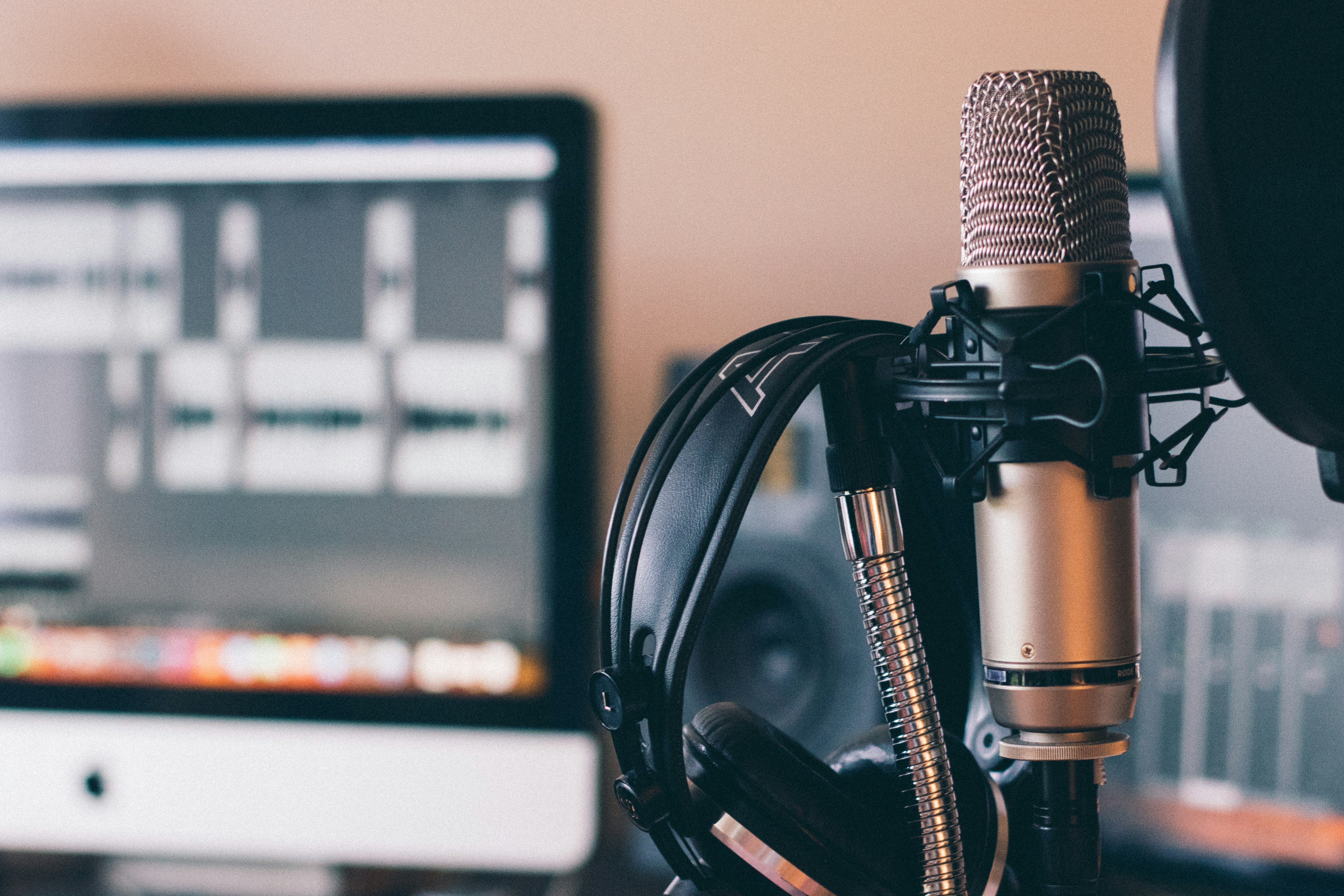
When we look back on the last couple years in college admissions, the issue of staff morale will be one of the hallmarks of the post-pandemic era. That's why I was excited to host this important conversation with Elizabeth Kirby about how - specifically - she was paying attention to this issue for her team.
The full conversation is available on demand: https://www.crowdcast.io/c/morale
Staff Morale

Understanding enroll ml and Outcome Optimization Theory in Enrollment Management
Marketers have long mastered optimizing the consumer journey by focusing on strategic touchpoints that drive long-term loyalty and value. It's time for enrollment management to harness that same power. After a decade of mixed results striving towards real-time predictive analytics in higher education admissions, we can now pose an interesting thesis: perhaps we may have been using the wrong tool for the job. This post introduces you to the concept of Enrollment Outcome Optimization, which is deployed by enroll ml to prioritize long-term outcomes over immediate predictions—empowering admissions teams to make better-informed, more impactful decisions. This approach leads to more consistent, data-stable results, fundamentally transforming the enrollment process.
What is Outcome Optimization?
Outcome Optimization allows admissions counselors to identify and guide best-fit students through the enrollment process strategically. Unlike traditional predictive analytics, which are like road signs requiring constant interpretation, Outcome Optimization provides a GPS-like path, highlighting the critical actions that influence a student’s decision to enroll. This approach helps counselors focus on meaningful interactions today while planning future steps, aligning efforts to support students' needs and boost enrollment success.
Key Benefits of Outcome Optimization:
- Strategic Decision-Making: Focuses on high-impact actions to keep best-fit students on the optimum enrollment path.
- Holistic View: Offers a comprehensive understanding of the student journey, from current status to future steps.
- Risk and Opportunity Identification: Detects deviations from expected behaviors, helping mitigate melt risks and capture incremental enrollments.
- Optimized Focus: Prioritizes high-value students, reducing time on low-impact interactions.
- Consistency and Efficiency: Streamlines prioritization, enhances resource use, and provides predictable outcomes.
- Proactive Engagement: Anticipates challenges and guides students toward their enrollment goals.
How enroll ml's Machine Learning Enhances Enrollment Strategies
enroll ml uses advanced machine learning to provide deep, actionable insights that transform how admissions teams approach enrollment:
- Complex Pattern Identification: Analyzes diverse data points to uncover hidden patterns signaling a student’s likelihood to enroll, disengage, or require intervention.
- Daily Re-Scoring: Continuously updates student scores based on the latest data, enabling timely, data-driven decisions.
- Clear Funnel Prioritization: Simplifies complex data into actionable priorities, ensuring focused strategies on high-potential students and melt risks.
Outcome Optimization vs. Traditional Predictive Analytics
- View of the Student Journey: Traditional analytics have a fragmented, short-term focus, while enroll ml's Outcome Optimization takes a holistic, long-term approach to key moments.
- Identifying Opportunity and Risk: Traditional methods are reactive and spread efforts thin, whereas Outcome Optimization proactively prioritizes high-interest students.
- Consistency in Outcomes: Predictive models can be inconsistent and reactive to data changes; Outcome Optimization replicates proven behaviors for consistent results.
- Decision-Making Approach: Traditional analytics react to current data points, while Outcome Optimization anticipates and addresses future challenges.
- Focus on High-Value Activities: Traditional models dilute focus across numerous signals; Outcome Optimization concentrates on impactful actions.
- Ease of Implementation: Predictive analytics often require complex, frequent recalibrations, while Outcome Optimization simplifies by focusing on critical moments.
Why Outcome Optimization is Essential in Today's Enrollment Landscape
Enrollment management has evolved with efforts like the Common App and direct admit programs, reducing barriers but complicating predictive models. Here's why Outcome Optimization is needed:
- Dilution of Signals: Traditional indicators like campus visits now suggest less genuine interest due to easier application processes.
- Increase in Data ‘Noise’: A surge in low-intent applicants creates significant noise, making predictive models less accurate.
- Changing Student Behavior: Minimal effort in exploring options has shifted behavior from strong interest to casual exploration.
- Limitations of Traditional Models: Predictive models lose effectiveness deeper in the funnel; real-time engagement provides a clearer picture of intent.
- Need for Transparency: Students benefit from transparent, tailored communications aligned with their demonstrated interests, fostering a more connected enrollment journey.
When is Outcome Optimization Most Effective?
- Real-Time Decision Making: Traditional methods may suffice, but enroll ml’s approach offers a more strategic perspective.
- High-Frequency Low-Value Transactions: Predictive analytics can be useful, but Outcome Optimization focuses on high-impact actions.
- Complex Strategic Processes, Identifying Key Success Factors, Consistency Over Time, Resource-Constrained Environments, Long-Term Strategic Planning, Behavioral Change in Teams: All these areas benefit more from Outcome Optimization due to its strategic focus and ability to maintain consistent results.
Conclusion
While traditional predictive analytics remain critical for market understanding and investment decision-making, enroll ml's machine learning-driven Outcome Optimization is better equipped for the complexities of daily enrollment management. By offering a strategic, holistic view, reducing fragmented efforts, and consistently focusing on high-value activities, enroll ml helps admissions teams achieve more consistent and impactful enrollment execution and outcomes.
Understanding the theory behind enroll ml's machine learning driven outcome optimization
.jpg)
The role of admissions teams in higher education has always been crucial, but it's becoming increasingly complex as institutions strive to enroll more students while managing limited resources. Traditional methods often leave admissions counselors bogged down with manual tasks, diverting their attention away from meaningful student interactions. Enter machine learning, a transformative technology that can significantly enhance decision-making and time management, boosting the overall efficiency of admissions operations.
Machine learning offers a game-changing solution by automating and optimizing various aspects of the admissions process. One of the most significant impacts is on the time management of admissions counselors. Studies show that counselors spend a significant portion of their time on data-related activities, which can be both time-consuming and monotonous. By integrating machine learning, these routine tasks can be automated, allowing counselors to reclaim over 30% of their time for more strategic activities.
For instance, machine learning models can sift through vast amounts of application data, identifying high-potential candidates based on behavioral patterns and engagement metrics. This automated analysis not only speeds up the process but also improves accuracy, ensuring that no promising student is overlooked. By highlighting the most relevant candidates, machine learning enables counselors to focus their efforts where they are needed most, enhancing the efficiency and effectiveness of their outreach.
Moreover, machine learning enhances decision-making by providing real-time insights and predictive analytics. Traditional methods often rely on historical data, which can quickly become outdated. In contrast, machine learning continuously processes new data, offering up-to-date insights that reflect the current enrollment landscape. This real-time analysis empowers admissions teams to make informed decisions swiftly, adapting their strategies to changing trends and student behaviors.
Another key benefit is the ability to personalize engagement with prospective students. Machine learning can analyze individual interactions and preferences, allowing admissions teams to tailor their communications and outreach efforts. Personalized emails, targeted follow-ups, and customized content resonate more with students, increasing their likelihood of enrolling. This targeted approach not only improves conversion rates but also enhances the overall student experience, making them feel valued and understood.
Furthermore, machine learning can identify and address potential issues before they escalate. For example, if a particular segment of students shows signs of disengagement, machine learning models can flag these patterns early, allowing admissions teams to intervene proactively. Timely interventions can significantly impact student decisions, turning potential drop-offs into successful enrollments.
The integration of machine learning also facilitates continuous improvement in admissions strategies. With each enrollment cycle, machine learning models learn and adapt, refining their predictions and recommendations. This iterative process ensures that admissions strategies remain effective and aligned with evolving student behaviors and institutional goals.
Ultimately, machine learning is a powerful tool that can revolutionize the efficiency and effectiveness of admissions operations. By automating routine tasks, providing real-time insights, and enabling personalized engagement, machine learning empowers admissions teams to make better decisions and manage their time more strategically. This not only improves enrollment outcomes but also creates a more dynamic and responsive admissions process. Embracing machine learning is essential for institutions looking to optimize their resources and stay competitive in the rapidly evolving landscape of higher education.
Comments